Main Second Level Navigation
Philip M. Kim
PhD
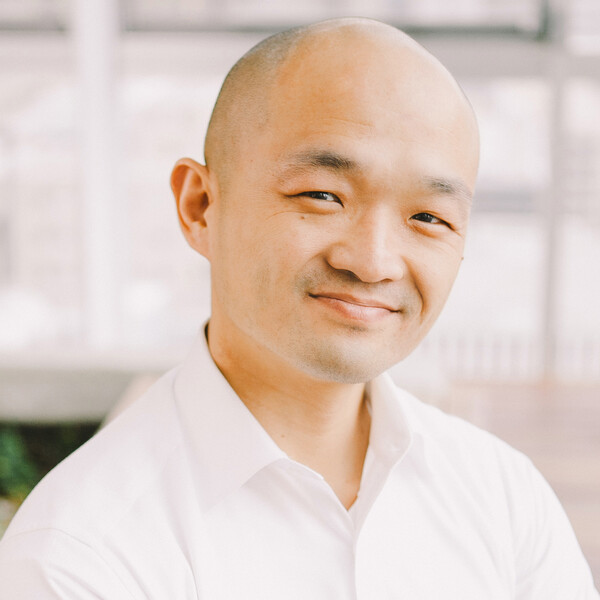
Qualification
- Yale University, New Haven, CT, U.S., Research Fellow in Molecular Biophysics and Biochemistry, 2005-2008.
- Massachusetts Institute of Technology, Cambridge, MA, U.S., PhD in Physical Chemistry / Artificial Intelligence, 1998-2003.
- University of Tuebingen, Germany, Vordiplom in Physics and Biochemistry, 1994-1997.
MY RESEARCH OVERVIEW (GO TO SCIENTIFIC OVERVIEW)
Integrating modern computational and experimental techniques to help develop novel therapies
Researchers are experiencing perhaps the most exciting period in biomedical science in history. Advances in experimental technologies are equivalent in scale to the innovations of the industrial revolution. For example, the speed and cost of genome sequencing have improved over 6 orders of magnitude! These advances make computational and theoretical methods front and centre in today’s era of biomedical research.
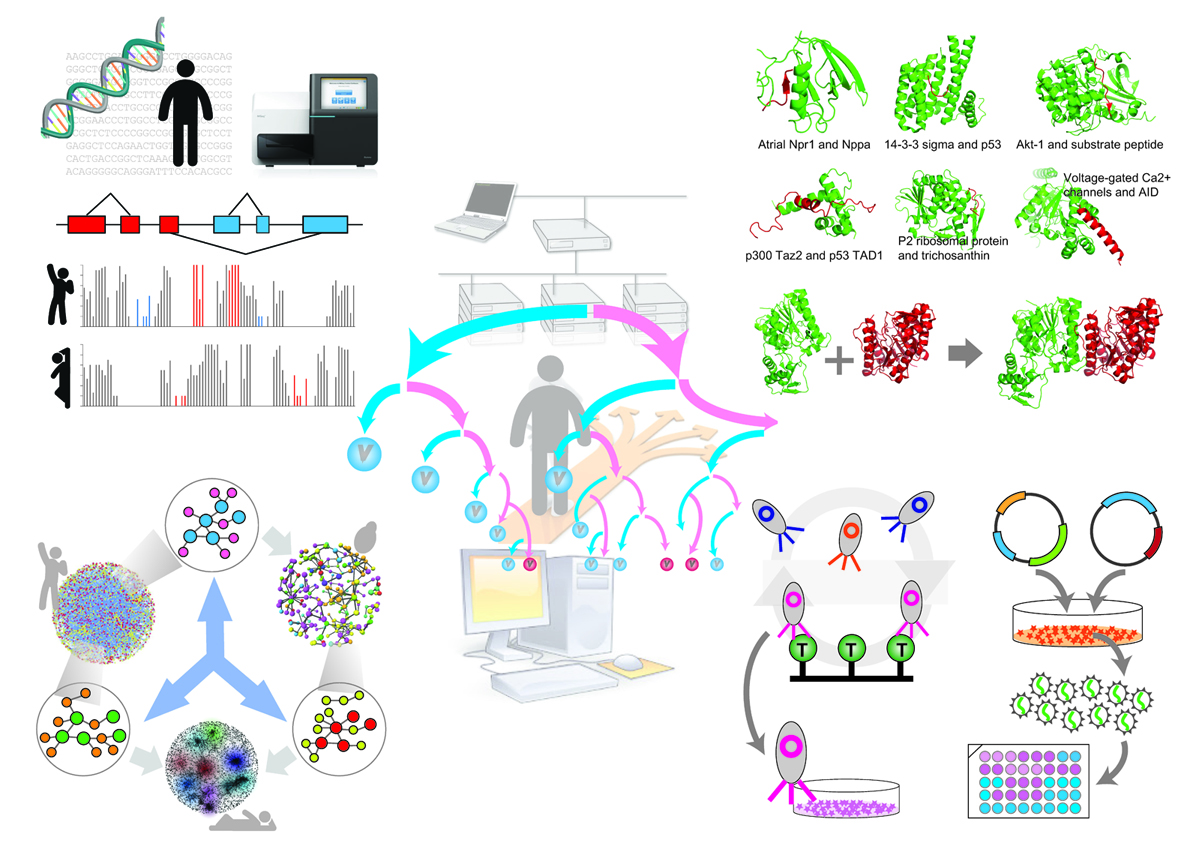
Our ultimate goal is to contribute to the development of novel therapeutics. The group focuses their efforts on studying proteins, their interaction networks and ways in which they can be exploited for drug discovery. Many drugs work by enhancing or disrupting these interactions so it is pivotal to understand the specificities of such protein-protein contacts.
To achieve their goals, our group integrates approaches from diverse fields such as computational biology, molecular biophysics, protein engineering and genomics. Research in our lab is uncovering fundamental rules that govern protein-protein interactions and is laying the groundwork for new avenues of drug discovery. If you want to think about personalized medicine, think computational biology first!
SCIENTIFIC RESEARCH OVERVIEW
Current projects in the lab include:
1. Predicting and validating novel combination therapies
As development of new drugs is a difficult challenge, some ‘low-hanging fruit’ is the use of existing therapeutics in new ways. One particularly promising venue is to combine them with other drugs to form new combination therapies. As there are millions of possible combinations, this requires the development of powerful machine learning algorithms to narrow the search space for experimental screening. We are currently developing such algorithms based on a variety of genomic, pharmacological and proteomics data and are linking it tightly to high-throughput screening to validate and refine our predictions.
2. Highly parallel intracellular inhibition of protein-protein interactions identifies novel cancer drug leads
Protein-protein interactions (PPIs) are emerging as a promising new class of drug targets. We are developing novel high-throughput approach to inhibiting PPIs in cells. We design proteome-wide libraries of human peptide binding motifs and use a pooled lentiviral system to intracellularly screen for their effects on cell proliferation. We thereby can identify peptides that have specific phenotypic effects. For instance we can screen for peptides leading to drastic reduction in cell viability in the malignant cancer cell lines while leaving non-malignant control lines unaffected (to find future cancer therapeutics), or we can conversely, find peptides that would lead to better survival of neurons (to find future therapeutics for neurodegenerative diseases).
We can then optimize such candidates for affinity and specificity using computational methods and test them both in vivo and in vitro. We are also working on different venues how to deliver such peptides to the cell.
3. Protein engineering via high-throughput selection of computational designs
Current combinatorial selection methods for protein engineering have successfully optimized protein scaffolds for binding against a range of protein targets. However, these methods are inherently limited by the employed library randomization strategies, whereby even large libraries only sparsely sample sequence space. Meanwhile, great advances in computational protein design have largely been underutilized. We thus work on developing integrated approaches to engineering high-affinity binders that first computationally design thousands of individual protein binders and then synthesize and select for the highest affinity designs in parallel. We have demonstrated the feasibility of our approach in a proof-of-concept study and successfully obtain low nanomolar binders. We have shown that our approach can work with a variety of selection methods, including in vitro methods such as phage display or cell based methods such as yeast-two-hybrid.
4. Structure-based protein interaction networks
It has been a long standing interest of mine to combine a structural (“bottom up”) view with a network (“top-down”) view of biology. Thanks to many recent advances, we are now able to generate proteome-wide structurally-resolved networks. We are using those models to help understand a variety of cellular phenomena. For instance, we are seeing a rapid increase in the number of catalogued mutations, many of which are associated with diseases. However, to draw mechanistic conclusions, a biochemical understanding of these mutations is necessary. We have thus introduced ELASPIC, a novel ensemble machine learning approach that is able to predict stability effects upon mutation in both, domain cores and domain-domain interfaces. We are now working to use further use these biophysical effects to understand the mechanisms behind the mutations phenotypes. We are further using structural interactions networks to understand the effects of alternative splicing on the protein level.
SELECT PUBLICATIONS
- Semi-supervised Learning Predicts Approximately One Third of the Alternative Splicing Isoforms as Functional Proteins. Hao Y, Colak R, Teyra J, Corbi-Verge C, Ignatchenko A, Hahne H, Wilhelm M, Kuster B, Braun P, Kaida D, Kislinger T, Kim PM. Cell Rep. 2015 Jul 14;12(2):183-910.
- Large-scale interaction profiling of PDZ domains through proteomic peptide-phage display using human and viral phage peptidomes. Ivarsson Y, Arnold R, McLaughlin M, Nim S, Joshi R, Ray D, Liu B, Teyra J, Pawson T, Moffat J, Li SS, Sidhu SS, Kim PM. Proc Natl Acad Sci U S A. 2014 Feb 18;111(7):2542-7.
- Distinct types of disorder in the human proteome: functional implications for alternative splicing. Colak R, Kim T, Michaut M, Sun M, Irimia M, Bellay J, Myers CL, Blencowe BJ, Kim PM. PLoS Comput Biol. 2013 Apr