Main Second Level Navigation
Gary Bader
PhD
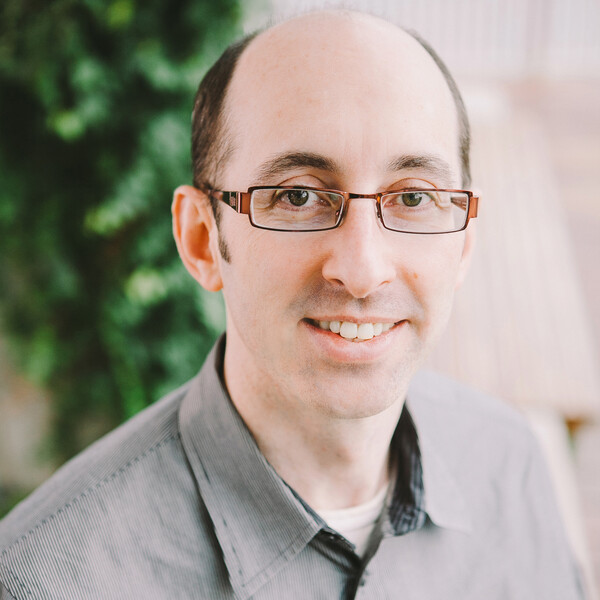
Qualification
- Memorial Sloan-Kettering Cancer Center, New York, NY, U.S., Research Fellow in Computational Biology, 2002-2006.
- University of Toronto, PhD in Biochemistry (Bioinformatics), 2002.
- McGill University, Montreal, BSc in Biochemistry, 1997.
OTHER AFFILIATIONS
- Lunenfeld-Tanenbaum Research Institute, Sinai Health System, Toronto.
- Department of Computer Science, University of Toronto.
MY RESEARCH OVERVIEW (GO TO SCIENTIFIC OVERVIEW)
Using a supercomputer to find disease causes and treatments
The human genome project has unveiled a large number of parts, but scientists don’t fully understand how these parts fit together. Revealing and understanding this information is important, as biomolecules interact inside us and arrange themselves into intricate networks and pathways that control all aspects of a cell’s function. Diseases arise if this network is broken in specific ways. Understanding exactly how this complicated network functions has the potential to improve diagnosis, prognosis and therapy and reduce the cost of medical care in Canada and the world.
Advances in genomic technologies allow scientists around the world to ‘look under the hood’ of our bodies like never before. They can identify the intricate pattern of genes that are turned on and off to run physiological systems and can map entire genomes of individuals. Scientists can do the same under disease conditions to see which parts of the system are acting differently when compared to healthy conditions.
This information provides an unprecedented opportunity to understand how diseases arise, but to do this, researchers need to assemble all of this information into a model of how cells function. Imagine taking millions of photos of buildings, cars and people over time and combining them into one blueprint of how a city works. Studying this blueprint may help identify how gridlock interferes with an otherwise smooth flow of traffic and suggest ways to fix the problem.
My lab develops computer-based analysis methods to tie all of the information we know about our cells into a blueprint of how our body works. Our methods have led to the discovery of biological systems underlying autism and the first potential targeted therapy for a childhood brain cancer. We are also developing software and computational technologies for open-access use by scientists around the world.
Visit Dr. Gary Bader's Discover Research profile to learn more.
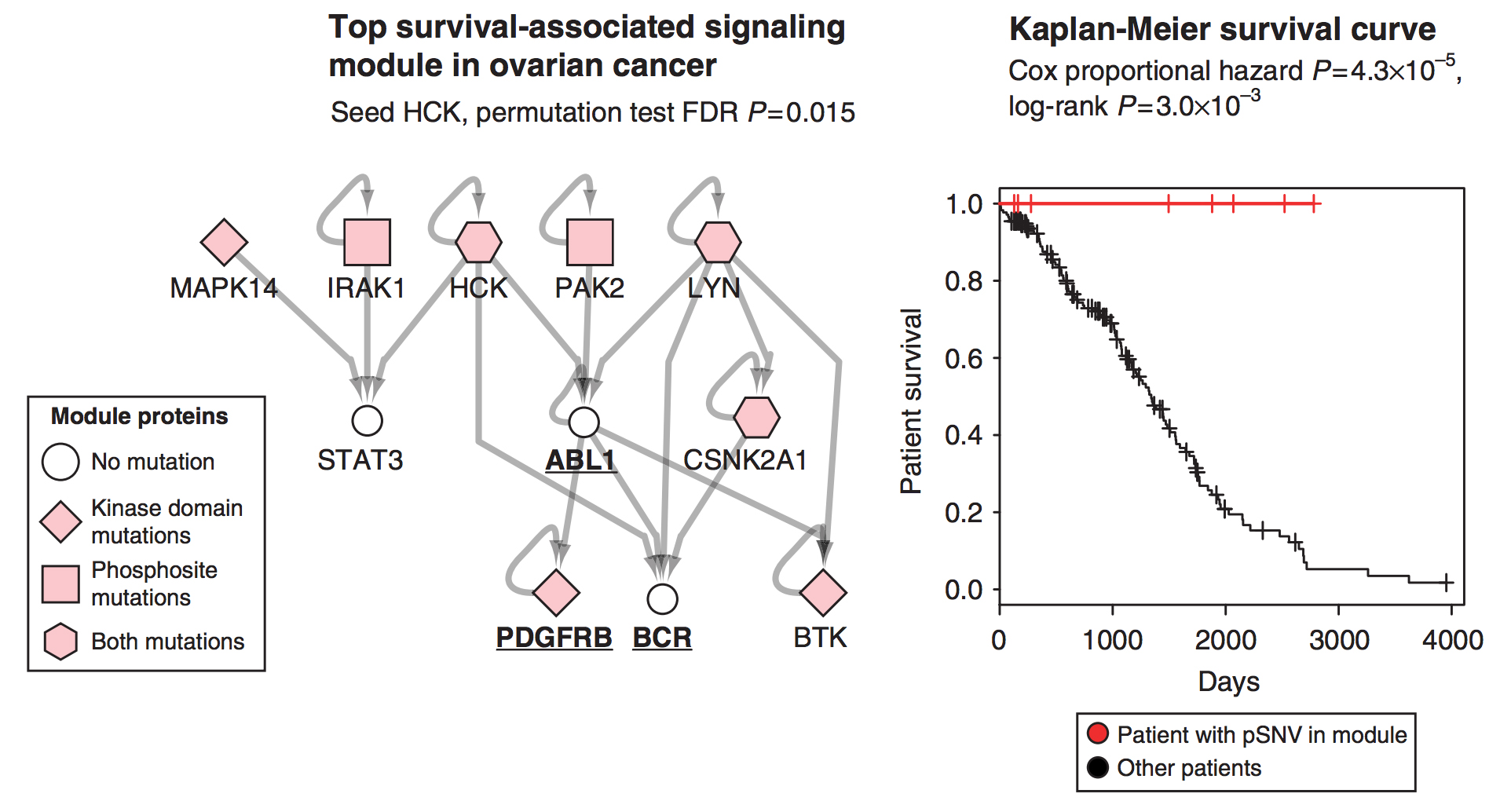
SCIENTIFIC RESEARCH OVERVIEW
We seek to better understand the relationship between genotype and phenotype using a model of how cells work (information about cellular mechanisms and pathways). This helps us predict the functional consequence of mutations, such as those that cause cancer and other diseases, gain insight into how diseases work, improve diagnostics and prognostics and identify new therapies. Our lab is mostly computational, but we frequently collaborate with wet lab and clinical groups.
The concept of using prior knowledge about cellular mechanisms (pathways, reactions, interactions) to study disease phenotypes has many benefits over traditional approaches to analyze genomic data at the individual gene or genomic region level. First, it improves statistical power in two ways: A) it aggregates counts of mutations across all of the genes and genomic regions involved in the given cell mechanism, providing a higher number of counts that makes statistical analyses more reliable; B) it reduces the dimensionality from tens of thousands of genes or millions of genomic regions (e.g. SNPs) to a much smaller number of ‘systems’ or ‘pathways’, thereby reducing the cost of multiple hypothesis testing. Second, results are often easier to interpret because the analysis is phrased at the level of familiar concepts such as ‘cell cycle’ or ‘protein phosphorylation site’. Third, the approach can help identify potential causal mechanisms and drug targets. Fourth, results obtained from related, but different, data may be more comparable since results are projected onto a smaller, shared feature space (i.e. a limited number of pathways). Fifth, the approach facilitates integration of diverse data types, such as genomics, transcriptomics and proteomics, to aid in identifying disease cause. In sum, projecting disease data onto known mechanisms increases statistical and interpretative power.
We have four research and development projects that work towards this goal.
1. Precision medicine
Our newest research project is to use ‘omics, clinical and cellular mechanism data to improve patient outcome by developing more precise diagnostic and prognostic systems. This involves identification of disease markers, disease subtypes and machine learning systems that predict patient outcome based on a wide variety of available patient data.
http://www.ncbi.nlm.nih.gov/pubmed/24713437
2. Active cell map
The 'active cell map' is the set of all interactions, complexes and pathways involving molecules in the cell and their activity under normal and diseased regulatory circumstances. We are developing novel computational methods to combine molecular network and ‘omics profiles to uncover active cell map regions. These are useful to gain mechanistic insight into diverse biological systems and conditions and to identify important drug targets. We successfully applied this concept to identify histone and DNA methylation by the PRC2 complex as the first rational therapeutic target for ependymoma, a common type of childhood brain cancer, in a project led by Dr. Michael Taylor. DNA methylation is targetable by known drugs, such as 5-azacytidine, which stopped rapid metastatic tumour growth when used on compassionate grounds in a terminally ill patient.
http://www.ncbi.nlm.nih.gov/pubmed/24553142
3. Genome to network mutation interpretation
We are developing computational methods to accurately predict the binding specificity of peptide recognition domains (e.g. SH3, SH2, protein kinase, WW, PDZ) given the domain sequence and to predict biologically relevant protein-protein interactions given binding specificity. This helps us predict how genome sequence changes affect the molecular network in the cell and in turn cellular and physiological phenotypes.
Review: http://www.ncbi.nlm.nih.gov/pubmed/22561014
4. Community software development
The Bader Lab is involved in a number of collaborative open-source bioinformatics projects designed to make biological pathway and network data easy to visualize and analyze.
SELECT PUBLICATIONS
- MIMP: predicting the impact of mutations on kinase-substrate phosphorylation. Wagih O, Reimand J, Bader GD. Nat Methods. 2015 Jun;12(6):531-3.
- Evolutionary constraint and disease associations of post-translational modification sites in human genomes. Reimand J, Wagih O, Bader GD. PLoS Genet. 2015 Jan 22;11(1):e1004919
- Single cell-derived clonal analysis of human glioblastoma links functional and genomic heterogeneity. Meyer M, Reimand J, Lan X, Head R, Zhu X, Kushida M, Bayani J, Pressey JC, Lionel AC, Clarke ID, Cusimano M, Squire JA, Scherer SW, Bernstein M, Woodin MA, Bader GD, Dirks PB. Proc Natl Acad Sci U S A. 2015 Jan 20;112(3):851-6