Main Second Level Navigation
Hannes Röst
PhD

Qualification
- Stanford University, Stanford, CA, U.S., Research Fellow in Personalised Medicine, 2015-2017.
- Swiss Federal School of Technology (ETH), Zurich, Switzerland, PhD in Computational Mass Spectrometry, 2014.
- Swiss Federal School of Technology (ETH), Zurich, Switzerland, MSc in Computational Biology and Bioinformatics, 2010.
- Swiss Federal School of Technology (ETH), Zurich, Switzerland, BSc in Biology, 2008.
MY RESEARCH OVERVIEW (GO TO SCIENTIFIC OVERVIEW)
Using computers to understand mass spectrometric data
The sequencing of the human genome has provided us with a molecular parts list of the human cell. The next challenge in biology is to understand what the function of each part is and how these parts fit together. Such an understanding will provide insights into how cells live and die, why they decide to grow or stop growing and how they process signals from the environment to make these decisions. By comparing healthy with disease cells, researchers can understand which parts malfunction and tell us where to look for potential cures for a disease.
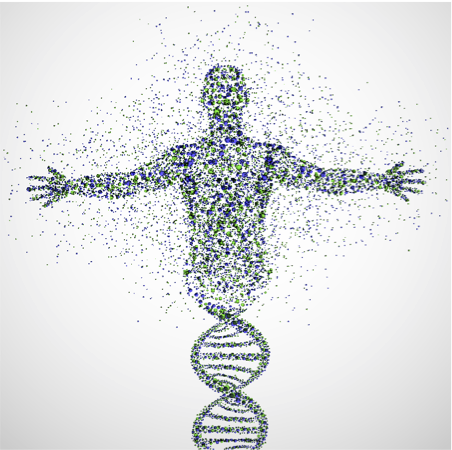
Scientists and doctors are increasingly using novel high-throughput methods to gain extremely detailed insights into the molecular causes for disease. By collecting millions of data points during each patient visit, they can gain a personalized understanding of the patient's disease risks and suggest patient-specific interventions, such as drugs or lifestyle changes. Performing such high-throughput analysis routinely in the clinic will allow doctors to observe, intervene and cure with much greater speed and accuracy, providing the right drugs to the right patients and detecting a disease before it has progressed too far.
However, tracking thousands of proteins and small molecules in every patient is currently too expensive and takes too long. We are working with next-generation mass spectrometric instruments to measure proteins and metabolites with unprecedented accuracy and throughput. These instruments can produce more measurement data in less time and our algorithms then interpret the wealth of data generated by these instruments. We develop software capable of analyzing millions of mass spectrometric scans and extract accurate quantitative information from this data. We collaborate with researchers and doctors around the world using our tools and algorithms, helping them to interpret mass spectrometric data or develop new ways to answer long-standing biological questions.
SCIENTIFIC RESEARCH OVERVIEW
Current projects in the lab include:
1. Personalized Medicine
Personalized medicine entails a vision where biomedical data is used to generate both static individual risk profiles (through personalized genomes) and to track patients' health longitudinally using dynamic molecular and physiological data. Mass spectrometry (MS) plays a critical role in providing quantitative molecular profiles of patients over time, allowing early detection of disease and monitoring of interventions. While MS-based proteomics and metabolomics technologies capture crucial dynamic information on the molecular level, they currently lag behind sequencing-based technologies due to a lack of throughput, reproducibility and coverage.
Personalized medicine is based on the premise of "biochemical individuality", i.e. the notion that individuals differ in their biochemical make-up and (i) may have different "normal" (healthy) levels of certain analytes and (ii) may react differently to external interventions and perturbations (such as lifestyle changes or medication). However, to understand the impact of protein and metabolite levels for health and disease on a personal level, we need to be able to track these analytes with mass spectrometry and be able to interpret their variation within the context of the inter-personal and intra-personal variation as well as the personalized genome.
2. Quantitative Proteomics and Metabolomics
Mass-spectrometry based quantitative proteomics allows researchers to accurately quantify the dynamics of protein abundance and protein activity in biological systems. In order to increase the quantitative accuracy and the throughput of proteomics methods, we have developed a novel targeted proteomics method called SWATH-MS that is based on data-independent acquisition (DIA) which aims to complement traditional mass spectrometry-based proteomics techniques such as shotgun and SRM methods. In principal, it allows a complete and permanent recording of all fragment ions of all peptide precursors in a biological sample and can thus potentially combine the advantages of shotgun (high throughput) with those of SRM (high reproducibility and sensitivity).
To analyze the SWATH-MS data, we developed OpenSWATH, an automated software to perform targeted data extraction from the SWATH-MS maps. Our software allows to perform automated data extraction, peak-picking and feature-detection in chromatographic traces, thus performing a complete SWATH-MS data analysis completely automatically; the only input are the raw MS/MS files as well as a transition library to perform the targeted data extraction. After feature detection, we use the mProphet algorithm for error rate estimation.
Using SWATH-MS in conjunction with OpenSWATH, we have successfully quantified over 900 proteins in the pathogen Streptococcus pyogenes in a single LC-MS/MS injection (more than any previous study), allowing us to study the response of the pathogen to human blood plasma in unprecedented detail. We also could quantify over 1900 human proteins in an AP-MS pulldown experiment and identify over 500 high-confidence physical protein-protein interactions of the 14-3-3β scaffold protein, giving us direct insight into the dynamics of a large protein interaction network.
3. Human Variation
We are interested in studying human variation in healthy subjects in collaboration with other research labs. The hPOP (Human Personalized Omics Profiling) project is designed to study the variance of molecular markers across a large number of participants. Recent advances in high throughput technologies allow profiling of thousands of analytes within a single experiment. These measurements could potentially be used to diagnose disease early, monitor treatment progression and stratify patient groups to ensure each individual obtains the treatment best suited to their needs. This personalized approach to medicine would include continuous monitoring of thousands of parameters over a whole lifetime. However, in order to be able to interpret such data, we need to have a better understanding of the underlying natural variation of these molecular parameters in health and disease. Only if we know the natural ranges of individual analytes, the expected responses to perturbations and the long-term trends in their levels, can we draw meaningful conclusions from comprehensive personalized profiling.
4. Simulation
Often, biological phenomena cannot be studied or measured directly (or doing so would be too resource-intensive) and researchers need to use in silico simulations to analyze complex phenomena. Some well-known examples in computational biology include protein folding or kinetic modelling where computer simulation-based approaches are used heavily. In mass-spectrometry based proteomics, peptide digestion, chromatographic separation and collision-induced dissociation to fragment charged peptide precursor ions are complex phenomena which can be approached using simulation to provide predictions and insights into error rates during peptide identification and peptide quantification.
Our software, the SRMCollider, allows to model all individual steps in a LC-MS/MS experiment (digestions, chromatographic separation, fragmentation), specifically taking into account the challenges of targeted proteomic where only a few fragment ions are monitored for each peptide. This allowed us to investigate the question of assay redundancy in SRM and SWATH-MS experiments and make concrete predictions about assay specificity in a targeted proteomics setting. We have successfully applied these simulations for multiple studies in the Aebersold lab, including proteomes as diverse as Mycobacterium tuberculosis, Saccharomyces cerevisiae and Homo sapiens.
SELECT PUBLICATIONS:
- OpenMS: a flexible open-source software platform for mass-spectrometry data analysis. Röst HL*, Sachsenberg T*, Aiche S*, Bielow C*, Weisser H*, Aicheler F, Andreotti S, Ehrlich HC, Gutenbrun-ner P, Kenar E, Liang X, Nahnsen S, Nilse L, Pfeuffer J, Rosenberger G, Rurik M, Schmitt U, Veit J, Walzer M, Wojnar D, Wolski WE, Schilling O, Choudhary JS, Malmström L, Aebersold R, Reinert K, Kohlbacher O. Nat Methods. 2016 Aug 30;13(9):741-8.
- TRIC: an automated alignment strategy for reproducible protein quantification in targeted proteomics. Röst HL, Liu Y, D’Agostino G, Zanella M, Navarro P, Rosenberger G, Collins BC, Gillet L, Testa G, Malmström L, Aebersold R. Nat Methods. 2016 Sep;13(9):777-83.
- OpenSWATH enables automated, targeted analysis of data-independent acquisition MS data. Röst HL*, Rosenberger G*, Navarro P, Gillet L, Miladinović SM, Schubert OT, Wolski W, Collins BC, Malmström J, Malmström L, Aebersold R. “OpenSWATH enables automated, targeted analysis of data-independent acquisition MS data.” Nat Biotechnol. 2014 Mar;32(3):219-23.