Main Second Level Navigation
Jesse Gillis
PhD
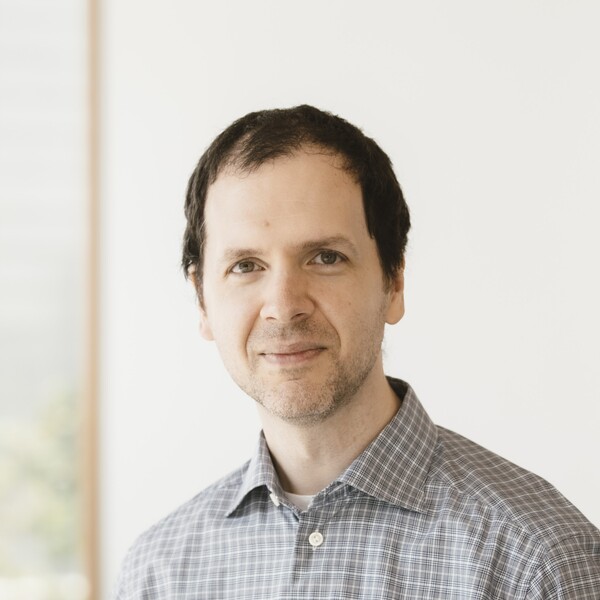
Qualification
- Cold Spring Harbor Laboratory, U.S., Associate Professor, Genomics, 2017-2021.
- Cold Spring Harbor Laboratory, U.S., Assistant Professor, Genomics, 2012-2017.
- University of British Columbia, Department of Psychiatry, Postdoctoral Fellow, 2007-2012.
- University of Toronto, PhD in Physiology, 2003-2007.
- University of Toronto, MSc in Physiology, 2001-2003.
MY RESEARCH OVERVIEW (GO TO SCIENTIFIC OVERVIEW)
Research in my lab aims to understand the flow of information from the genome to whole organism biology through modeling and analysis of functional genomics data. This research is broadly integrative across modalities, systems, and even species but also integrative across levels of organization, using molecular processes within cells to understand how and why cells diversify and how that diversity in turn affects organism phenotype.
As functional genomics data has continued to increase in abundance and specificity, my lab has benefited strongly from the opportunities to provide organizing frameworks, deeply grounded in both biology and statistical insight. We have been particularly interested in determining base vocabularies to compare quite disparate data with the goal of better exploiting conservation as a central principle to understand function in physiological systems. For example, by comparing human data to that from other species, we are able to establish which genomic features robustly contribute to variation at the molecular, cellular, and organismal level.
SCIENTIFIC RESEARCH OVERVIEW
Neuronal cell types
Characterizing neuronal cell types is a crucial step towards understanding how neurons work together and how neurological disorders arise. To this end, NIH’s BRAIN Initiative Cell Census Network (BICCN) consortium published a collection of articles providing an unprecedented atlas of neuronal cell types across a wide variety of modalities and species (single-cell transcriptomics, epigenomics, spatial transcriptomics, connectivity, morphology, electrophysiology).
One of the Gillis lab’s interests within the BICCN is to quantify the replicability of these novel cell types across laboratories and modalities. We developed a statistical tool called MetaNeighbor that uses a neighbor voting system to identify cell types with consistent expression signatures, suggesting broad support across a variety of experiments and computational pipelines. Cell types with high MetaNeighbor scores are more likely to replicate in independent studies, thus more likely to be observed and easier to target in follow-up experiments. Thanks to its flexibility and scalability, MetaNeighbor was successfully used in a variety of contexts, from the characterization of novel cell types in maize to the understanding of evolutionary convergence and divergence of neurons across mammals.
Signatures of individuality
Cells in a fully developed organism share life histories traced back through their divisions, defining lineages. Marks left on a cell early in the lineage can be inherited down through cell divisions, leaving shared features across cells, barcoding their lineages. Attempts to ascertain the existence of these permanent shared markings in previous work have mainly focused on the strongest events – monoallelism – in the simplest systems – cell lines – with mixed results. However, like X-inactivation, lineage specification occurring via autosomal epigenetic marks may have a very broad impact across both the genome and cell populations.
In order to more systematically assess their organismal impact, we turned to Dasypus novemcinctus (the nine-banded armadillo) which has a polyembryonic reproductive strategy, producing litters of identical quadruplets. This system enables an unusual level of environmental and genetic control, allowing us to assess cellular barcoding arising as noise in the assignment of epigenetic marks on alleles. In combination, these allelic imbalances progressive barcode trajectories across cell lineages as they move forward during development. We show that autosomal allelic ratios varying between individuals, and consistent with an early developmental original, are also enriched for stability over time, suggesting this is likely to have an important impact on disease variability by rendering otherwise haplosufficient genetic variation penetrant.
Cross-kingdom Co-expression
During evolution, species often acquire new functions and structural features that help them adapt to their surroundings. Changes in gene regulation across species is one of the common mechanisms underlying this functional evolution. Since co-expressed genes reflect shared function, comparative cross-species analysis of gene coexpression is useful for inferring essential, evolutionarily conserved regulatory modules, as well as species-specific expression changes driving morphological novelty.
Using meta-analytic coexpression networks, we investigate the evolution of gene regulation in 37 species across the eukaryotic tree of life. Ancient genes are conserved across kingdoms, while younger genes show cell type and species-specific expression profiles, suggesting new function acquisition through specialized expression signatures. This framework has also enabled us to analyze and compare the evolution of functional modules in organisms with poor genetic annotation, elucidating essential systems and separating them from niche metabolic pathways. By leveraging this powerful method and applying it across the plant kingdom, we can efficiently evaluate thousands of gene modules for their conservation, identifying deeply conserved metabolic modules and revealing modules being rapidly rewired towards new functions. We find that secondary metabolism modules are frequently repurposed for novel functions across species, and that in comparison to animals, plant primary metabolism genes develop novel functions much more rapidly.
X Chromosome variability
Mammalian females are effectively composed of two genetically distinct cellular populations that vary in expression from the X-chromosome via X-Chromosome Inactivation (XCI). XCI is a stochastic, permanent, and developmentally early epigenetic decision made in every female cell to transcriptionally silence a single X-allele to match the single X-chromosome in males. Taking into account the inherent stochasticity and permanence of XCI, shared variance in XCI mosaicism across cellular populations is indicative of shared developmental lineage. We have developed methods to model X-linked allelic imbalance from transcriptomics data, allowing us to quantify variance in X-linked mosaicism across human tissues, individuals, and across mammalian species. By extracting and comparing variance in X-linked mosaicism across tissues, individuals, and species, we aim to identify fundamental features of lineage and conserved developmental principles.
Variance in XCI additionally plays an important role in heterozygous X-linked disease phenotypes in females, where the direction and degree of allelic inactivation can directly influence disease phenotypes. X-linked chronic granulomatous disease (X-CGD) is a rare, monogenetic primary immunodeficiency characterized by recurrent, life-threatening infections and the formation of phagocyte-derived granulomas at infection loci. Comparing single-cell expression profiles between cells expressing the wildtype or mutated allele for X-CGD within a single carrier tightly controls for the impacts of genetic variation in disease pathogenesis. We aim to apply single-cell transcriptomic approaches to X-CGD carriers to identify important cell autonomous signaling in X-CGD pathogenesis.
Gene Function Learning
Because correlated gene expression implies involvement in shared processes, coexpression is a powerful, albeit noisy, source of information about function. This informs several aspects of our lab's activities. To address inter-/intra-experiment variability and noise we aggregate many datasets to build coexpression networks (Lee,J. et al. 2020). To make expression data as easy to use and intepretable as sequence-based data, we develop efficient network algorithms to perform various kinds of inference and to test replicability (Ballouz,S. et al. 2017; Fischer,S. et al. 2021). As part of our effort to demonstrate the utility of aggregate expression networks, we participated in fourth Critical Assessment of Function Annotation (CAFA), demonstrating that the performance of the combination of expression and sequence-based data is better than either alone.
Coexpression data is especially useful when combined with other data modalities and when generated cross-species. Our lab has generated aggregate Hi-C chromatin contact networks combining up to 100 datasets per species. HiC and expression networks were then probed in combination, demonstrating improved performance of trans- interactions measuring evolutionary conservation and divergence. Aggregate meta-networks can be used as input, individually or in combination, to more complex machine learning frameworks to explore gene function, regulation, and evolution. Planned work along these lines will leverage expression data with Google DeepMind's AlphaFold to probe protein-protein interactions.
SELECT PUBLICATIONS
- Single-cell co-expression analysis reveals that transcriptional modules are shared across cell types in the brain. Harris, B.D., Crow M., Fischer S., Gillis J. Cell Systems. 2021 May, ISSN 2405-4712.
- Predictability of human differential gene expression.Crow M., Lim N., Ballouz S., Pavlidis P., Gillis J. Proc Natl Acad Sci U S A. 2019 Mar, ISSN 0027-8424.
- Characterizing the replicability of cell types defined by single cell RNA-sequencing data using MetaNeighbor.Crow M., Paul A., Ballouz S., Huang Z. J., Gillis J. Nature Communications. 2018 Feb, 9 (1). p. 884. ISSN 2041-1723.